Statistics Tutorials: All You Need to Know About Hypothesis Testing: The Tricks You Need to Learn
Hypothesis testing can be a confusing topic, especially if you don't know the foundations well. By learning a couple of easy of principles, you will be able to understand all that there is to know about hypothesis testing.
You can learn it best by practicing on your own, or with a proficient stats tutor who can guide you through.
What is a Hypothesis Test?
That the first question we will address. A Hypothesis test is a statistical procedure that uses sample data to make a decision about a certain claim, that involves a certain population parameter. So, the actors required for conducting a hypothesis test are:
(1) The sample data
(2) A certain claim about a population parameter
Without any of the two above, can test an hypotheses. Now, let us go a bit further and explain what are those two main components
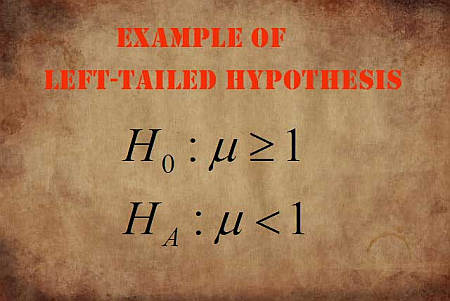
What is the method of hypothesis testing? The sample
Let us recall that a sample is a smaller subset of a whole population. And, a population is the complete set of subjects that you want to investigate about.
Typically, the populations are large, so if we want to make a statement about a large population, we try to do so by selecting a small sample, in hopes that the sample will somehow carry information about the whole population. That seems to be a long shot, but it turns out to be true in some cases.
Our hope is that by analyzing a small sample from a population, we will be able to know a lot about the population. When that happens, we say that the sample is representative of the whole population. But not just any sample will do.
We need to collect something called a random sample. There are different strategies for collecting random samples, depending on the type and size of the population, but what I want you to retain now is that there are somewhat reasonable procedures to produce random samples, which are expected to be representative of their populations.
And, once you have a random sample, you will be using a procedure using hypothesis testing which will help you to get information about the whole population from the sample.
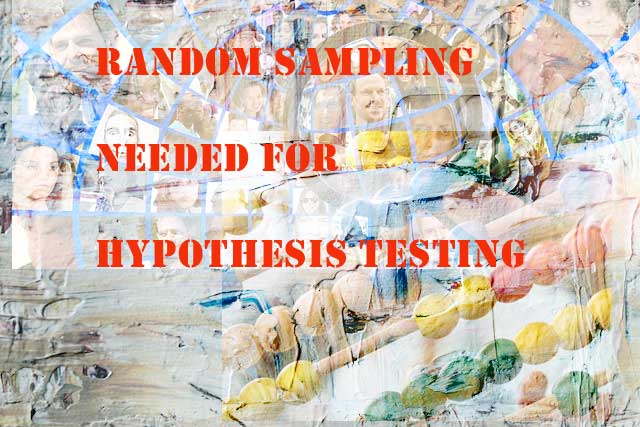
What is hypothesis testing and its purpose? A Claim About a Population Parameter
Now that you have a sample, you need a claim to test. There are good and bad news. The good news are that population parameters are simple numbers, so that a claim about a population parameters is simply about what could the potential value of that population parameter be. What I mean by this is that claims are very simple from a structural point of view.
For example, assume that you a random variable that is normally distributed, with an unknown mean equal to \(\mu\). We would like to take a sample of that population and say something about \(\mu\). The claims about \(\mu\) are claims about its potential values. I mean, something like \(\mu =10\) is an actual claim, or \(\mu <10\) is a claim as well. Anything stating a possible set of values for a population parameter is a claim.
The bad news is that we cannot test just any claim. In order to conduct a hypothesis test and test a claim about a population parameter, we need to have certain structure. Namely, we can only work with two types of claims, or in this context, we need to define between two hypothesis: the null hypothesis and the alternative hypothesis.
These two hypotheses are both claims about a population parameter, with peculiarity that (a) they must not overlap and (b) the null hypothesis must contain the "=" sign in it.
Let me rephrase that: If you want to run a hypothesis test you must have two hypothesis, the null hypothesis and the alternative hypothesis. These two hypotheses are both claims that are stating something about the numerical value of the population parameter.
The set of potential values of the population parameter that are stated in the null hypothesis CANNOT have any value in common with the set of potential values of the population parameter that are stated in the alternative hypothesis. Also, the null hypothesis must contain the sign "=" in its algebraic statement. For example, \(\mu =13\) and \(\mu \le 13\) are examples of null hypotheses, but \(\mu >10\) cannot be a null hypothesis.
What is hypothesis testing with example?
A null hypothesis is written as \({{H}_{0}}\) and a alternative hypothesis is written as \({{H}_{A}}\). An example of a properly defined set of hypothesis is
\[\begin{align} & {{H}_{0}}:\mu =10 \\ & {{H}_{A}}:\mu \ne 10 \\ \end{align}\]But, for example, this set of hypotheses is not valid:
\[\begin{align} & {{H}_{0}}:\mu =10 \\ & {{H}_{A}}:\mu \ge 10 \\ \end{align}\]Why the above set is not valid? Because the set of possible values stated by \({{H}_{0}}\) and \({{H}_{A}}\) overlap (see that both null and alternative hypotheses include 10 as a possible value for \(\mu\)).
The Mechanics of a Test of Hypothesis
Now that you have a sample and you have a properly defined null and alternative hypotheses, you can conduct a test of hypothesis. Now you can compute a test statistic, that is the center piece of the whole process.
A test statistic is simply a numerical (random) value that is computed from the sample data and from the values stated in the hypothesis. The actual formula used to compute a test statistic depends on the type of parameter being estimated (for example, we use a different type of test statistic when we are testing for a population mean \(\mu\) than when we are testing for a population variance \(\sigma\)).
The philosophy, though, for ALL hypothesis test is the SAME. Please retain this in your head: The test statistic is computed and its outcome is checked assuming that the null hypothesis is true.
What are the 4 hypothesis testing steps?
So the principle is: If I assume that the null hypothesis \({{H}_{0}}\) is true, how unlikely are the same results obtained? The philosophy is that if the sample results is to unlikely under the assumption that \({{H}_{0}}\) s true, then we discard \({{H}_{0}}\) as a plausible option.
The probability that the sample results are at least as extreme as the ones observed can be typically computed (because usually assuming that \({{H}_{0}}\) is true determines the value of the unknown parameter that determines the distribution of the population), and this probability is called the p-value.
A low p-value indicates the sample results are unusual if we take \({{H}_{0}}\) as true. But, how low is low enough? Well, we need to define a threshold, which we call significance level, or \(\alpha\). This value of \(\alpha\) represents the risk we are willing to take of rejecting a true null hypothesis.
Results of a Hypothesis Test
So finally, how do we give our answer to the hypotheses? Simple, if the calculated p-value is such that $p<\alpha $, we then reject the null hypothesis. Otherwise, if \(p\ge \alpha\), we fail to reject the null hypothesis. Observe that there is no such thing as "accepting the null hypothesis". Sample data CANNOT prove the null hypothesis because of the fundamental way it is constructed.
If the null hypothesis is not rejected, the sample data is telling us "look, it does not seems that the sample data contradict the null hypothesis, so let us retain it, for now at least".
On the other hand, if the null hypothesis is rejected, the sample data is telling us "look, the sample data seems to be conflicting with the null hypothesis, so it'd be wise to check your null hypothesis, because it may be off".
Did We Get it Right?
One misconception is that a hypothesis test will give an infallible answer. It cannot be further from the truth. The decision about the hypothesis test (either reject Ho OR not reject Ho) can be actually wrong. Face the fact, go over it.
How can you be wrong? Actually, in two ways: First, if you reject the null hypothesis you will be claiming that the null hypothesis is not true. So, if the null hypothesis ACTUALLY true, you have then made an error. That is called a Type I error, in which your decision of rejecting Ho is wrong, because Ho is actually true. The probability of this type I of error is \(\alpha\).
The second type of error occurs when you fail to reject the null hypothesis, so then you don't find enough evidence to claim that the null hypothesis is false. But, if it turns out that the null hypothesis is ACTUALLY false, you have then made an error. This is called a Type II error, in which your decision of no rejecting Ho is wrong, because Ho is actually false. The probability of this type II of error is named as \(\beta\).
What is an example of hypothesis testing in real life?
There are so many examples of hypothesis testing in real life. For example, a stock market analyst may want to compare the market returns for the periods 1933 - 1980, and 1980 to present, and she may hypothesize that the population mean returns for both periods differ significantly.
Or for example, an education council manager may want to assess whether the education reform implemented in 1993 has had a significant effect on the results on standardized test for eight grades, for which using hypothesis testing can be useful in real life.
What if you need help
One of the best statistics homework help service you will find online is My Geeky Tutor, with 20 years online successfully helping students.
That is it for now.
|
Submit your problems for a free quote and we will be back shortly (a couple of hours max). It costs you NOTHING to find out how much it would cost to solve your problems.
We provide a quality problem solving service on the following stats topics:
- Probability
- Basic Concepts: Sample Space, Events.
- Densities and Distributions.
- Descriptive statistics.
- Descriptive Analysis of data.
- Graphs and charts.
- Inferential Statistics
- Means, variances, populations, samples.
- Intervals of Confidence.
- Z-test, T-test and F-tests.
- Hypothesis Testing.
- ANOVA.
- Correlation.
- Linear and non-linear regression.
- Non-parametric Statistics.
- Sign Test.
- Wilkinson Tests.
- Kruskal-Wallis Test.
- Spearman Correlation Coefficient.
Our team is highly experienced in SPSS, Minitab, EXCEL and the majority of the statistical software packages out there. Request your free quote. We a have a satisfaction guarantee policy. If you're not satisfied, we'll refund you. Please see our terms of service for more information about the satisfaction guaranteed policy. See also a sample of our work.
Why we can help with your Stats?
Experience
We have successfully helped customers online for more than 10 years now
Statistics Expertise
We can do handle any type of statistics analyis/homework/questions. Our tutors have real expertise, and big majority of our customers are returning customers
Step-by-Step Solutions
We provide detailed, step-by-step solutions, and we strive to provide exactly what our customers want.
Free Quote
E-mail us your problems, we will review them and promptly come back to you with a free quote
Very Competitive Prices
We strive to provide the best possible prices for our services
We take pride of our work
Our tutors take pride on the work we do. We diligently do work for our customers, and put great attention to details striving to always provide a great final product